Mutf_In: Nipp_Indi_Flex_Fpecv2
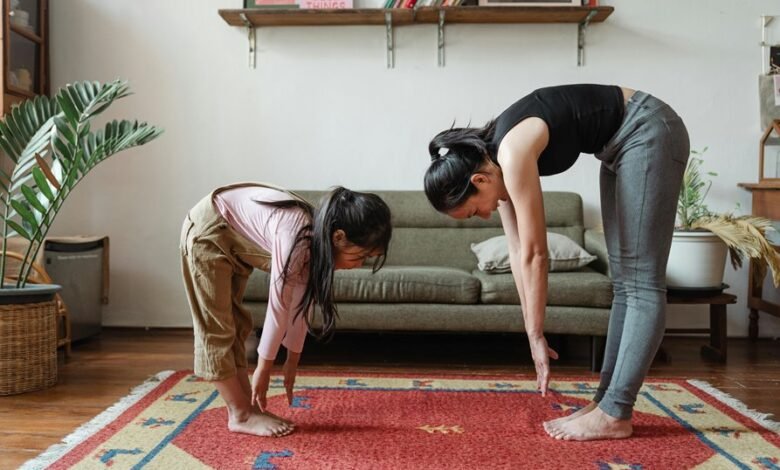
Mutf_In: Nipp_Indi_Flex_Fpecv2 represents a significant advancement within the Mutf_In framework. It enhances flexibility and adaptability in data processing through a modular architecture. Key innovations focus on optimizing performance and improving data flow. Moreover, it addresses critical issues surrounding data quality and ethical considerations. The implications of these developments for the fields of machine learning and data analytics warrant closer examination. What challenges and opportunities lie ahead in this evolving landscape?
Overview of Mutf_In Framework
The Mutf_In framework serves as a pivotal structure within the realm of data integration and processing.
Its architecture promotes modularity and scalability, allowing for efficient data handling across diverse systems.
Key benefits include enhanced operational flexibility and improved performance metrics, facilitating seamless data flow.
Organizations leveraging Mutf_In can achieve greater autonomy in managing their data ecosystems, aligning with the growing demand for adaptable solutions.
Key Features and Innovations
Key features and innovations within the Mutf_In framework significantly enhance its capability to address contemporary data integration challenges.
Its modular design facilitates flexible architecture, allowing users to tailor components for specific needs. Coupled with advanced performance optimization techniques, Mutf_In ensures efficient processing of diverse datasets.
These attributes empower organizations to adapt swiftly to evolving data landscapes, fostering greater analytical freedom and responsiveness.
Applications in Machine Learning
Applications of the Mutf_In framework within machine learning demonstrate its versatility and effectiveness in enhancing predictive analytics and data-driven decision-making.
By integrating advanced data preprocessing techniques, Mutf_In optimizes data quality, thereby improving model performance. Additionally, its robust model optimization strategies facilitate the fine-tuning of algorithms, leading to more accurate predictions and actionable insights.
Ultimately, this empowers users to harness the full potential of their data.
Future Directions and Impact
As advancements in technology continue to evolve, the future directions of the Mutf_In framework are poised to significantly influence the landscape of data analytics and machine learning.
Future advancements may enhance scalability and integration capabilities, yet potential challenges such as data privacy and algorithmic bias must be addressed.
Balancing innovation with ethical considerations will be crucial for sustainable development in this field.
Conclusion
In conclusion, the Nipp_Indi_Flex_Fpecv2 iteration of the Mutf_In framework emerges as a timely response to the increasing complexities of data processing. Coincidentally, as organizations face mounting pressures around data privacy and ethical considerations, this innovative version not only enhances performance but also aligns with the evolving demands of responsible data usage. Its advanced features promise to unlock new possibilities in machine learning, ultimately shaping a future where data-driven insights are both powerful and ethically grounded.